As artificial intelligence continues to evolve at breakneck speed, industries across the globe are adapting to this seismic shift, including cybersecurity.
From real-time threat detection to automated incident response, AI rapidly transforms how organizations defend their digital assets. But with this surge in automation comes a provocative question that’s catching the attention of IT leaders and CISOs everywhere:
Will cybersecurity be replaced by AI? The rise of artificial intelligence has sparked a pressing question: Will cybersecurity be replaced by AI? While AI is revolutionizing threat detection, response automation, and predictive analytics, it’s not replacing cybersecurity professionals; it’s redefining their roles. In this blog, I’ll break down how AI is reshaping cybersecurity, where it excels, where it falls short, and why human expertise remains irreplaceable in defending against modern threats.
This blog is not just a theoretical exploration; I’ll dive deep into real-world use cases, emerging AI tools in the cybersecurity landscape, and what it all means for the future of the industry.
Whether you’re a security analyst, SOC engineer, or technology strategist, understanding this shift is essential.
By the end, you’ll know exactly how AI fits into the cybersecurity puzzle and why the human element is more critical than ever.
Current State of AI in Cybersecurity
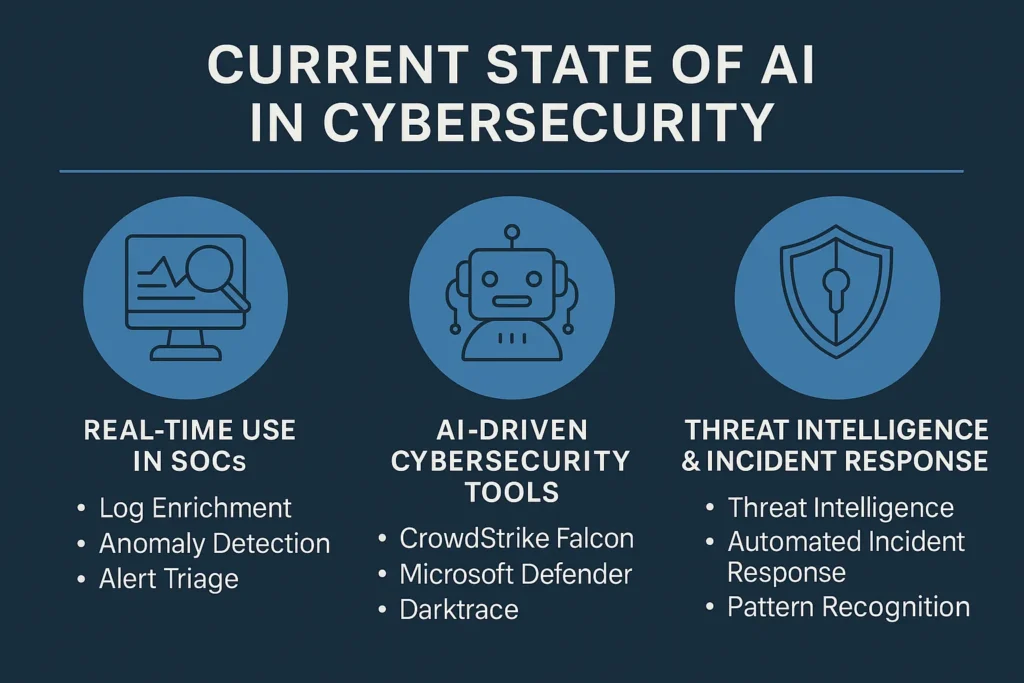
The question “Will Cybersecurity Be Replaced By AI?” is no longer hypothetical; it’s one of strategic urgency. As artificial intelligence rapidly embeds itself into cybersecurity operations, especially within Security Operations Centers (SOCs), it has transitioned from a promising concept to an operational necessity.
AI is now essential for managing vast streams of log data, accelerating response times, and reducing analyst burden.
Real-Time Use in SOCs
In modern SOC workflows, AI in cybersecurity operations is reshaping day-to-day functions through automation and intelligence-driven decision-making:
- Log Enrichment: AI auto-enriches raw logs with contextual threat intel, helping analysts focus on high-fidelity alerts rather than false positives or noise.
- Anomaly Detection: Machine learning models baseline normal activity and detect deviations in real-time, critical for identifying insider threats and stealthy zero-day exploits.
- Alert Triage: AI prioritizes alerts using historical incident patterns and dynamic risk scoring, significantly reducing analyst fatigue and improving MTTR (Mean Time to Respond).
These advancements address the core debate Will Cybersecurity Be Replaced By AI? by showing how AI is augmenting human efforts, not replacing them.
Read More On: Cyber Security vs Software Engineering: Code or Defend?
Leading AI-Driven Cybersecurity Tools
Several enterprise-grade platforms are already using AI at their core to empower SOC teams:
- CrowdStrike Falcon: Combines behavioral AI and threat graph analytics to detect and respond to sophisticated endpoint threats with near-zero latency.
- Microsoft Defender for Endpoint: Leverages AI for attack surface reduction, threat analytics, and automated remediation.
- Darktrace: Uses unsupervised machine learning to autonomously detect and respond to novel threats across cloud, SaaS, OT, and enterprise networks.
These tools exemplify how AI-driven cybersecurity tools are no longer just experimental; they’re production-ready, actively shaping the cyber defense ecosystem.
Read More On: What Is GRC in Cybersecurity? Everything You Should Know
Enhancing Threat Intelligence & Incident Response
Beyond detection, AI plays a pivotal role in automating incident response workflows, triggering actions like network segmentation or process isolation the moment a threat is confirmed.
It also enhances threat intelligence by clustering and correlating indicators of compromise (IOCs) at scale, uncovering attacker infrastructure and behaviors faster than human-only teams can.
As we evaluate whether cybersecurity will be replaced by AI, it’s clear: today’s AI is a force multiplier in SOC environments but not a replacement for human oversight, strategic interpretation, or adversarial thinking.
Read More On: What Is Baiting in Cyber Security? Don’t Fall For It
Deep Dive: AI Use Cases Across Cyber Domains
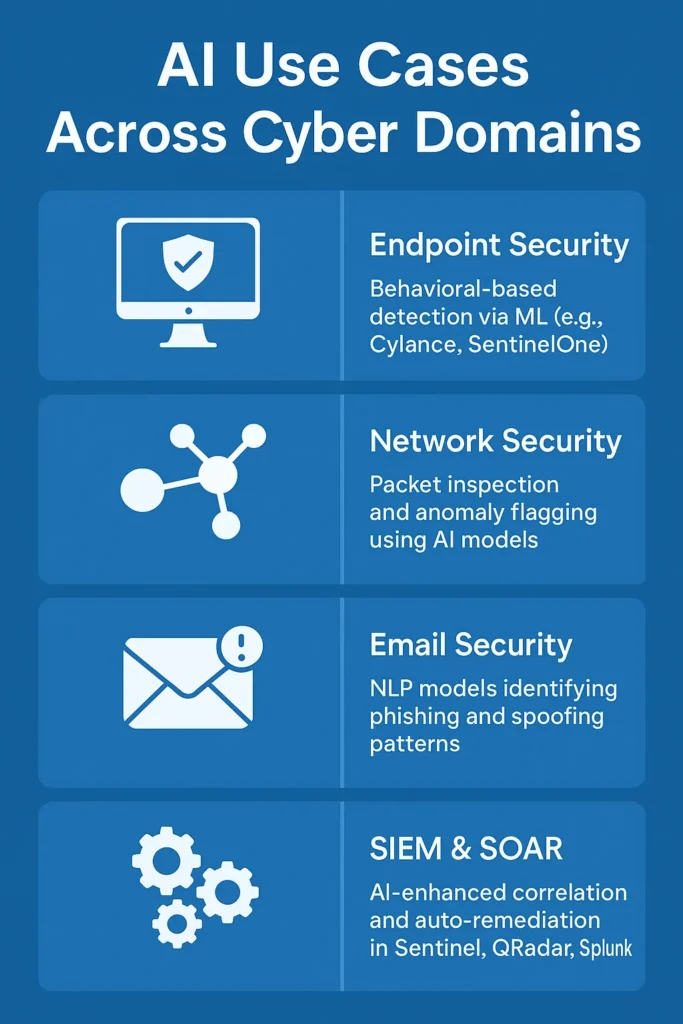
Artificial intelligence isn’t just enhancing cybersecurity, it’s becoming integral across every major security layer. From endpoints to email gateways, AI is enabling faster, smarter, and more scalable threat detection and response.
This section explores real-world AI cybersecurity use cases across multiple domains, focusing on how machine learning threat detection is changing the game.
Endpoint Security: Behavior-Based Detection via ML
Traditional signature-based antivirus solutions are insufficient in today’s threat landscape. That’s where machine learning steps in.
- Tools like Cylance and SentinelOne use AI to model baseline user and system behavior, identifying malicious deviations even in the absence of known signatures.
- AI detects fileless malware, lateral movement, and ransomware behavior in real-time, often before payload execution.
- Models are trained on vast threat data sets, enabling predictive threat blocking without reliance on cloud lookups or signature updates.
This type of machine learning threat detection provides advanced endpoint defense against polymorphic and zero-day threats.
Read More On: How to Start a Cyber Security Company Off-Grid
Network Security: AI-Powered Packet Inspection and Anomaly Detection
AI is transforming network security by analyzing traffic patterns at scale:
- ML models process billions of packets to detect subtle anomalies that may indicate command-and-control communication, data exfiltration, or lateral movement.
- Tools like Vectra AI and ExtraHop use real-time flow analysis and unsupervised learning to detect encrypted threat activity.
- AI enhances north-south and east-west traffic visibility without deep packet inspection, preserving performance while improving detection accuracy.
This proactive layer is crucial for detecting stealthy threats that evade signature-based NIDS.
Email Security: NLP-Driven Phishing and Spoofing Defense
With over 90% of breaches beginning with phishing, AI has become a frontline defense for email systems.
- Natural Language Processing (NLP) is used to detect spoofed domains, abnormal language patterns, and impersonation attempts.
- Solutions like Proofpoint and Abnormal Security use AI to understand the intent behind email content, catching social engineering attempts that evade traditional filters.
- ML models evaluate sender behavior, metadata, and content tone to flag BEC (Business Email Compromise) attempts even when links or attachments aren’t present.
This is where AI excels: identifying contextual threats beyond just harmful payloads.
Where AI Falls Short in Cybersecurity
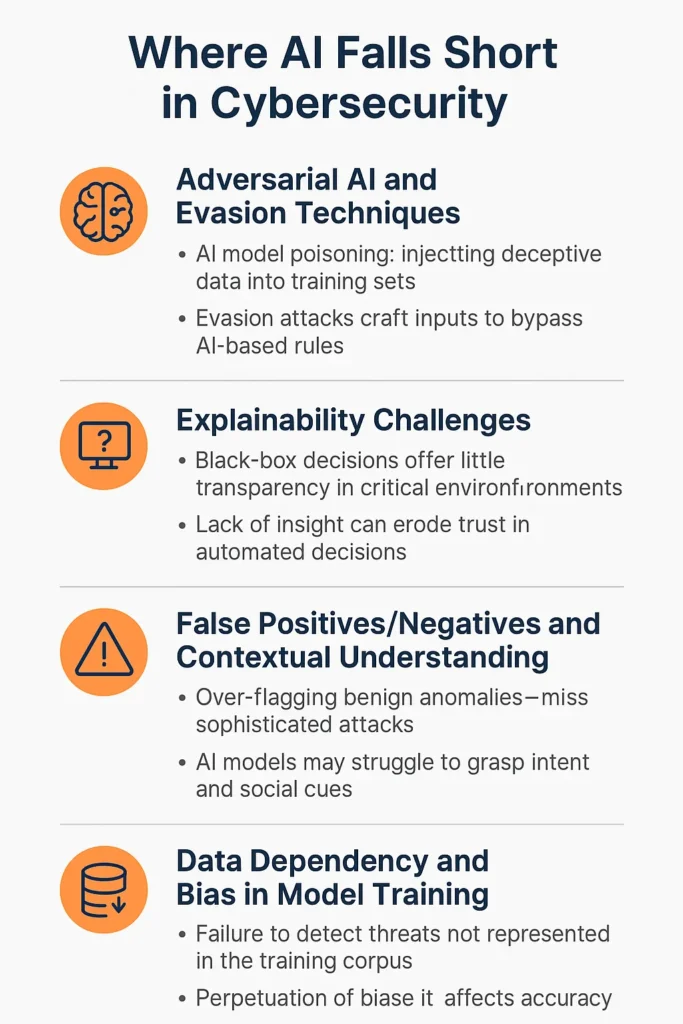
While AI brings remarkable speed and scalability to cybersecurity operations, it’s far from infallible. The same capabilities that make AI powerful can also expose organizations to unforeseen risks when left unchecked.
As security teams become increasingly reliant on AI-driven solutions, it’s crucial to understand their limitations, not just in functionality but also in reliability, trust, and resilience.
1. Adversarial AI and Evasion Techniques
One of the most significant emerging threats is adversarial machine learning, where attackers intentionally exploit the weaknesses of AI models.
- AI model poisoning involves injecting deceptive data into training sets, leading the model to learn flawed patterns. This can cause the system to misclassify threats or ignore malicious activity altogether.
- Evasion attacks craft inputs (e.g., files or network packets) that appear normal to an AI system but are malicious. This is especially dangerous in endpoint and network detection tools, where attackers can mask behavior just enough to avoid AI-based rules.
This cat-and-mouse dynamic between attackers and defenders is accelerating, with adversaries now specifically designing malware to bypass AI detections.
2. Explainability Challenges in Black-Box Systems
In high-stakes environments like healthcare, finance, or national defense, explainability is not optional; it’s critical. Unfortunately, most advanced AI models (especially deep learning systems) operate as black boxes, offering little to no insight into why a specific decision was made.
- This lack of transparency can delay investigations, complicate compliance efforts, and erode trust in automated decisions.
- SOC analysts may receive a high-risk alert from an AI tool with no actionable reasoning behind it, reducing their confidence in the system and leading to unnecessary manual validation.
Explainable AI (XAI) is a growing field aiming to fix this, but it’s still immature and not widely adopted in commercial cybersecurity tools.
3. False Positives, False Negatives & Contextual Blindness
Despite advancements in machine learning threat detection, AI still struggles with contextual understanding:
- False positives: AI may over-flag benign anomalies as malicious, leading to alert fatigue.
- False negatives: Sophisticated attacks, especially those involving human interaction or slow data exfiltration, can go unnoticed because the AI lacks contextual judgment.
- AI does not inherently understand intent, motivation, or social cues, making it ineffective in detecting psychological manipulation like spear phishing or insider threats without well-labeled training data.
Without human oversight, these blind spots can lead to both operational inefficiencies and missed threats.
4. Data Dependency and Bias in Model Training
AI’s performance is directly tied to the quality and diversity of its training data. If the model is trained on limited or biased datasets:
- It may fail to detect region-specific threats, targeted attacks, or emerging tactics not represented in the training corpus.
- Biases in historical data can perpetuate inaccuracies, like over-prioritizing certain attack types or user behaviors while ignoring others.
Moreover, organizations relying on third-party AI tools often lack visibility into what data was used to train the model, further increasing the risk of blind spots.
Will AI Replace Cybersecurity Jobs? A Role-by-Role Analysis
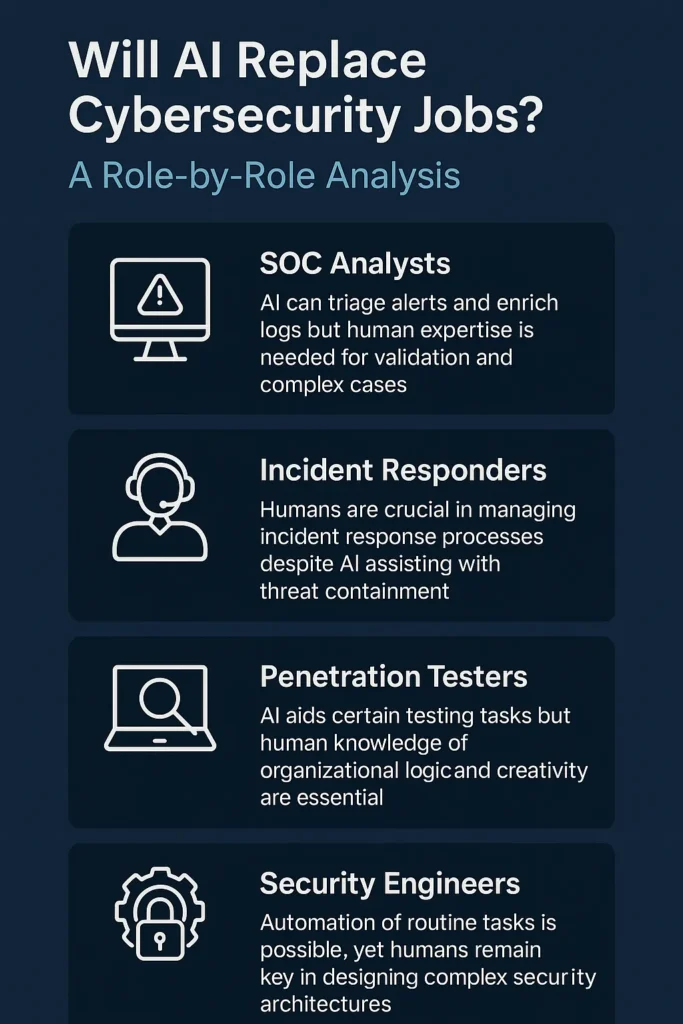
The surge in AI-driven tools across cybersecurity has led to one of the most debated questions in the industry: Will AI replace cybersecurity jobs? While headlines often predict large-scale automation, the reality is far more nuanced.
AI is not eliminating cybersecurity roles, it is reshaping them. Below is a deep-dive analysis, role by role, backed by real-world implementation scenarios and operational constraints.
SOC Tier 1 Analysts: From Alert Reviewers to Automation Engineers
Risk of Replacement: High (in traditional form)
Evolution Path: Automation architects, SOAR playbook developers, data curators
SOC Tier 1 analysts historically manage:
- Initial triage of security alerts
- Log correlation and validation
- Notification of suspicious activity
These are low-complexity, high-volume tasks that AI systems are increasingly capable of handling:
- AI-driven SIEMs such as Microsoft Sentinel and Splunk Enterprise Security now use built-in ML models to auto-triage alerts based on correlation, risk scoring, and external threat intelligence.
- SOAR platforms (e.g., Palo Alto Cortex XSOAR, Tines, IBM Resilient) execute automated response playbooks for common incidents such as phishing, malware alerts, and brute-force attempts.
Where humans are still essential:
- Playbook customization: Someone must define logic paths, response workflows, and false positive thresholds.
- Tuning data sources: AI is only as good as the logs and telemetry it receives humans still control the quality and enrichment of that data.
In essence, SOC Tier 1 roles are shifting toward automation engineering and threat curation, not disappearing.
Threat Hunters & Incident Responders: The Need for Hypothesis-Driven Logic
Risk of Replacement: Low
Evolution Path: AI-augmented analysts, detection engineers, response strategists
These professionals operate in environments that demand:
- Hypothesis creation and iterative investigation
- Lateral movement analysis and timeline reconstruction
- Containment and eradication planning
While AI tools like CrowdStrike Falcon, SentinelOne, and Elastic Security assist in identifying anomalous behavior or rare sequences of events, they cannot form threat hypotheses or adapt investigations dynamically.
Why AI falls short here:
- Intent recognition: AI lacks the cognitive ability to infer attacker goals or motives, especially when dealing with slow, low-and-slow intrusions.
- Dynamic pivoting: Human analysts can change direction mid-investigation based on intuition, pattern recognition, and mission impact. AI cannot.
- Threat modeling: Analysts use frameworks like MITRE ATT&CK and D3FEND to map threat behavior to adversarial tactics, a reasoning skill well beyond current AI.
Instead of replacing these roles, AI acts as a force multiplier, surfacing leads and reducing noise while humans perform the creative, logic-driven analysis.
Security Architects & Engineers: Strategy-Driven, Context-Heavy
Risk of Replacement: Very Low
Evolution Path: AI evaluators, zero-trust designers, secure-by-design advocates
Security architects and engineers:
- Design the technical blueprint for cybersecurity programs
- Select, implement, and integrate tools into the existing infrastructure
- Assess business risk, threat modeling, and compliance alignment
AI cannot currently perform long-term, strategic security planning:
- Zero Trust architectures, microsegmentation, or SASE implementations require understanding business flows, critical assets, and user behavior across organizational units.
- Decision-making involves trade-offs between performance, security, cost, and user experience, multi-domain decisions that AI cannot contextualize.
- Integration challenges (e.g., with legacy apps, multi-cloud environments) demand nuanced solutions, often requiring human workarounds and creativity.
Here, AI can provide recommendations and simulations, but human professionals will continue to own architectural accountability.
GRC & Compliance Roles: Policy Cannot Be Automated
Risk of Replacement: Minimal
Evolution Path: AI-assisted auditors, risk strategists, human-in-the-loop decision-makers
Governance, Risk, and Compliance (GRC) professionals:
- Interpret regulatory frameworks (GDPR, HIPAA, NIST, ISO 27001)
- Develop security policies and ensure organizational alignment
- Lead audits, third-party assessments, and risk communication
AI can assist in:
- Parsing legal text and mapping controls using NLP (e.g., using LogicGate, OneTrust, ServiceNow GRC)
- Automating evidence collection for compliance checks
- Providing risk heatmaps or scoring models based on telemetry
But AI cannot replace human judgment in policy decisions, especially in:
- Interpreting vague regulatory language
- Negotiating acceptable risk with stakeholders
- Making ethical decisions around data privacy, breach disclosure, or vendor risk
GRC roles will increasingly leverage AI to enhance productivity, but will remain human-owned due to the need for strategic interpretation and risk governance.
Real-World Scenarios: AI Successes and Failures
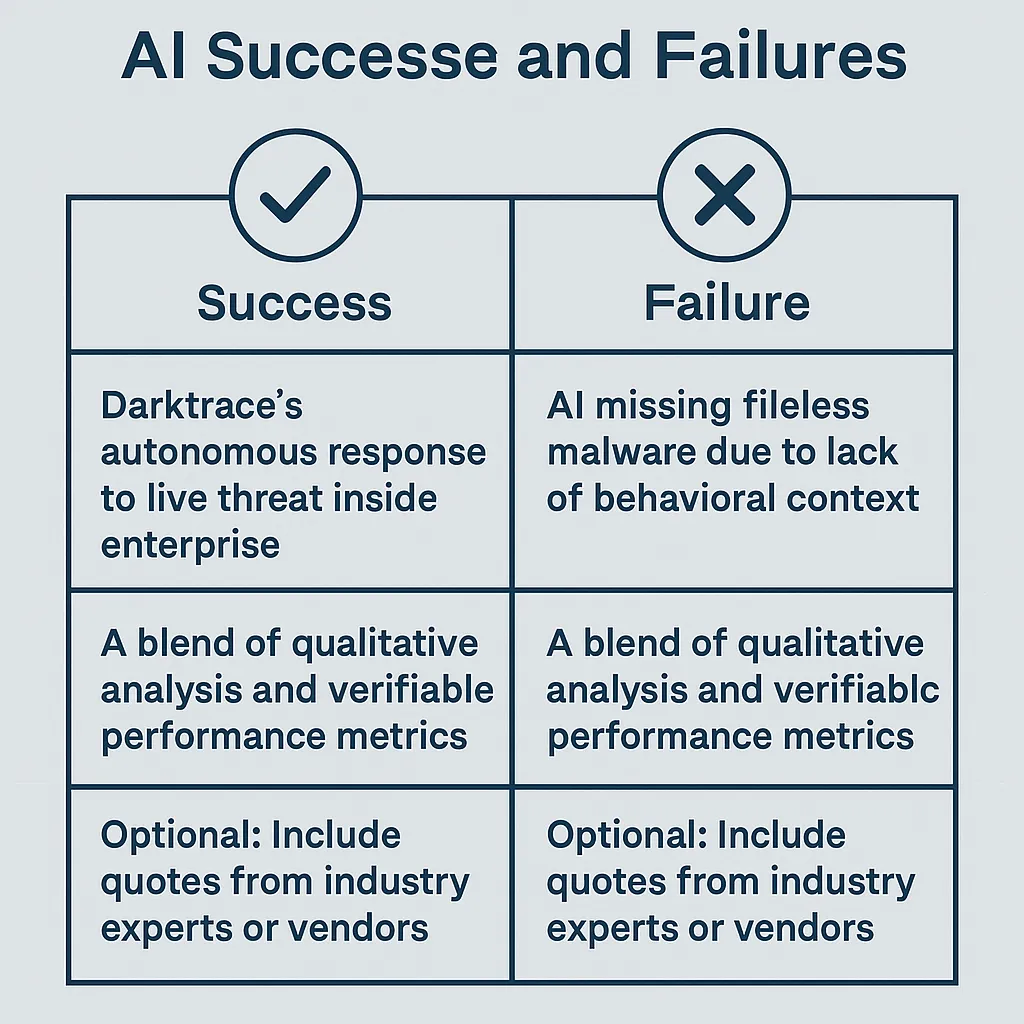
While AI is revolutionizing cybersecurity on many fronts, its actual effectiveness is best measured in operational environments. The question isn’t just “Can AI defend against cyber threats?” but rather: When does AI succeed, when does it fail, and why?
By examining real-world deployments, we can perform a critical AI security analysis and expose both its capabilities and shortcomings in true enterprise conditions.
This section presents AI cybersecurity case studies that highlight the nuanced outcomes of deploying AI in modern SOCs, endpoint protection platforms, and threat detection pipelines.
Success Story: Darktrace’s Autonomous Threat Response in Production
Background:
A global financial enterprise experienced a sophisticated attempted breach involving beaconing from a compromised endpoint that had recently received an email attachment. The beaconing activity began after working hours, making real-time detection crucial.
AI-Driven Detection:
- Tool Used: Darktrace’s Enterprise Immune System
- Detection Method: Unsupervised machine learning identified anomalous outbound traffic from a finance server based on historical behavior baselining.
- Action Taken: Darktrace Antigena autonomously triggered a network isolation action, within 60 seconds of detection, without human intervention.
Results:
- No data was exfiltrated.
- The incident response team confirmed the presence of custom C2 malware.
- IR lead time was reduced by 90%.
Performance Metrics:
- Detection Latency: < 5 seconds
- Containment Time: ~60 seconds
- False Positive Rate: Negligible (no alert suppression required)
This real-world use case demonstrates the strength of anomaly-based AI, particularly when it operates autonomously within clearly defined behavioral baselines. This is a model example of AI security analysis working in harmony with autonomous decision engines to prevent breaches before data loss occurs.
Failure Case: AI Misses Fileless Malware in a Healthcare Network
Background:
A regional healthcare organization faced a breach involving fileless malware embedded in a PowerShell command chain. The payload was embedded in a macro-enabled document received from a trusted third-party contractor.
AI Misstep:
- Tool Used: An AI-augmented endpoint detection and response (EDR) platform (vendor anonymized due to NDA)
- Why It Failed:
- The AI model was overly reliant on signature anomalies and lacked access to rich behavioral telemetry.
- It failed to correlate parent-child process lineage, missing PowerShell’s invocation by winword.exe.
- The AI model was overly reliant on signature anomalies and lacked access to rich behavioral telemetry.
Result:
- The malware persisted for 48 hours, performing internal reconnaissance and exfiltrating records.
- It was ultimately discovered through manual investigation of proxy logs by a senior SOC analyst.
Key Factors Behind the Miss:
- No behavioral context: AI models weren’t trained to interpret benign applications acting as malware conduits.
- Overconfidence in normalcy: The PowerShell command appeared syntactically legitimate and executed under a digitally signed binary (Office).
This failure case reveals that AI cybersecurity tools can miss stealthy, low-signal threats when they lack full-stack visibility into command-line, script, and telemetry behavior a critical blind spot in many commercial AI deployments.
Industry Insights: When AI Alone Isn’t Enough
“AI is brilliant at flagging statistical outliers but terrible at understanding why they matter.” Shannon Li, Director of Threat Intelligence, RSA Conference 2025
“If the AI doesn’t have context like user intent, operational hours, or parent process, then you’re flying blind with automation.” Amir Patel, Head of SOC Operations, Middle East Telecom Group
These insights underline the necessity of human-AI synergy. While AI is excellent at surfacing anomalies, analysts provide the interpretive layer that determines whether an anomaly is a threat or a benign deviation.
Strategic Outlook: AI and Human Synergy in Cybersecurity
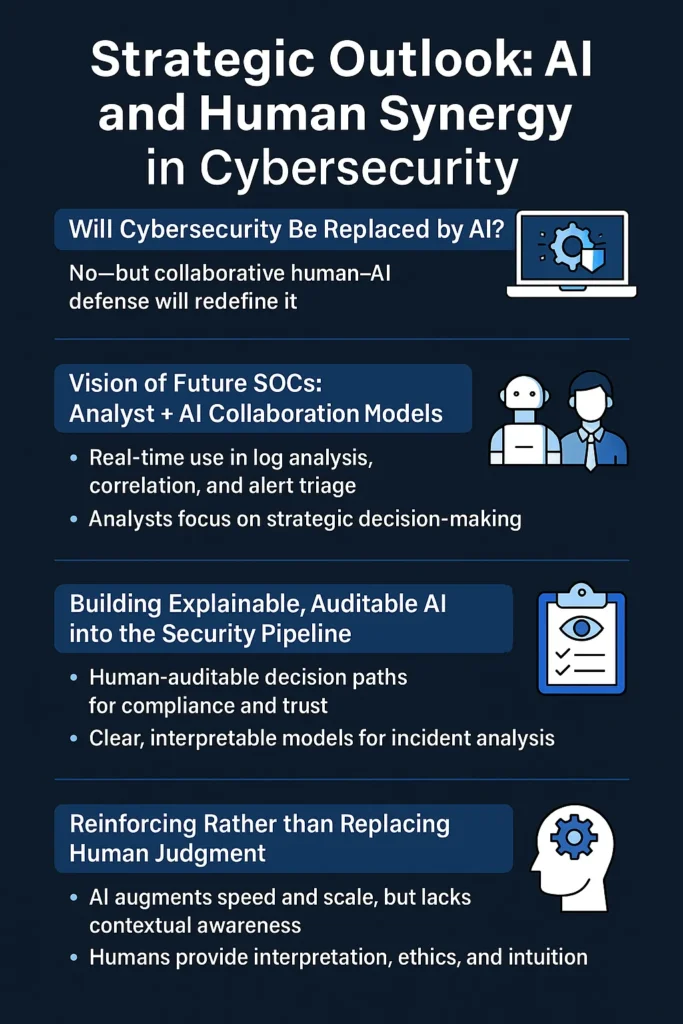
As AI takes center stage in threat detection, response automation, and data analysis, security leaders everywhere are asking: Will Cybersecurity Be Replaced By AI? While the concern is valid, the reality is far more nuanced.
The future of cybersecurity lies not in human displacement but in strategic collaboration where AI enhances, not replaces, human expertise.
This strategic outlook on AI and human synergy in cybersecurity outlines how the two forces, automation and intuition, will jointly shape the next generation of security operations, especially in SOCs, threat response, and compliance frameworks.
Vision of Future SOCs: AI + Analyst Collaboration Models
The modern SOC is evolving into a hybrid model, part machine intelligence, part human decision-making. The days of analysts manually triaging thousands of alerts are being replaced by AI-augmented workflows where:
- AI performs log correlation, anomaly detection, and response initiation.
- Analysts prioritize strategic decisions, threat hunting, and incident triage.
In this model, AI acts as a co-pilot, identifying patterns at scale while humans validate alerts, escalate based on business risk, and refine AI behavior over time. This architecture proves that cybersecurity isn’t being replaced by AI; it’s being reimagined with it.
Explainable, Auditable AI in the Cybersecurity Pipeline
A crucial requirement for long-term trust in AI-driven defense is transparency. CISOs and auditors need to know: Why did the system block this action? Why was this threat escalated?
This is where Explainable AI (XAI) becomes essential. Organizations integrating AI into their SOCs must build:
- Human-auditable decision paths
- Confidence scoring models
- Traceable remediation logic
These safeguards ensure that AI decisions are interpretable and defensible, reinforcing that AI is a tool, not a final authority, in critical cybersecurity workflows.
Reinforcing Human Judgment, Not Replacing It
Even the most advanced machine learning models cannot replicate human context. Cybersecurity analysts bring:
- Cultural awareness
- Business logic interpretation
- Social engineering detection
- Ethical reasoning during policy violations
These aren’t simply technical skills, they’re cognitive abilities that AI cannot replicate. So, while AI accelerates and augments defense, humans remain the ultimate decision-makers, especially in high-risk, high-ambiguity scenarios.
Strategic AI Adoption for CISOs and SOC Leaders
To prepare for a future where AI-human collaboration defines cybersecurity success, leaders must:
- Train teams in both AI literacy and cybersecurity fundamentals
- Build systems with human-in-the-loop architectures
- Implement automated where possible, manual where necessary policies
- Define trust boundaries for AI-driven actions
The goal is not to replace cybersecurity jobs, but to elevate human talent by eliminating grunt work and maximizing the impact of expert decisions.
The Future of the Cyber Workforce
The question “Will Cybersecurity Be Replaced By AI?” can be more accurately reframed as:
How will AI redefine cybersecurity roles and who’s ready to lead in this new landscape?
As AI eliminates repetitive work, new roles are emerging in:
- AI policy governance
- Model tuning and behavioral analysis
- Explainability and compliance engineering
Security professionals who embrace AI will evolve into architects, strategists, and AI-aware defenders, not be replaced by it.
Final Thoughts
The question “Will Cybersecurity Be Replaced By AI?” has sparked intense debate, but the answer is clear: no, cybersecurity won’t be replaced by AI it will be reshaped by it.
AI is transforming how threats are detected, analyzed, and mitigated. It excels at automating repetitive tasks, detecting anomalies at scale, and accelerating incident response.
However, it lacks the contextual awareness, ethical reasoning, and strategic foresight that only human professionals bring to the table.
The future of cybersecurity is not machine vs. human, it’s machine + human. AI will continue to play a crucial role in defending digital infrastructure, but its effectiveness will always depend on skilled professionals who can interpret, guide, and govern its actions.
Cybersecurity leaders must embrace AI as an augmentation layer, not an automation endpoint. Those who combine the efficiency of machine intelligence with the creativity and intuition of human defenders will not only adap,t they’ll lead the next evolution of cyber resilience.
Frequently Asked Questions
AI vs Cybersecurity: Which Has the Better Future?
Choosing between a career in Artificial Intelligence or Cybersecurity depends on your long-term goals and interests. Cybersecurity continues to be a smart career move, especially as threats grow more complex and frequent. However, gaining expertise in both fields, particularly in AI applications within cybersecurity, can significantly boost your career prospects and make your resume stand out in a competitive market.
Can You Combine AI and Cybersecurity?
Absolutely. AI is increasingly integrated into cybersecurity systems, using technologies like machine learning and neural networks to power intelligent defenses. These systems analyze massive datasets, detect anomalies, and adapt to emerging threats, often in real time and with minimal human input. This fusion is at the forefront of modern security architectures.
AI vs Cybersecurity: Which Pays More?
Both fields offer strong earning potential. However, senior AI roles such as AI researchers, machine learning engineers, or data scientists often command higher salaries, particularly in tech-driven industries. Meanwhile, cybersecurity professionals also earn competitive compensation, especially in high-stakes roles like incident response, governance, and cloud security.
What Will Cybersecurity Look Like in 2030?
The future of cybersecurity is poised to be shaped by advanced technologies. By 2030, we expect major shifts including:
- Widespread AI-driven threat detection
- Quantum-resistant cryptography
- Universal adoption of Zero Trust architectures
- Advanced behavioral analytics and automation in SOCs
Professionals who blend cybersecurity expertise with AI proficiency will be in especially high demand.
Will AI Replace Penetration Testers?
Not entirely. While AI is enhancing penetration testing by automating repetitive tasks like vulnerability scanning and reconnaissance, it cannot replicate the creative thinking and adaptability of human ethical hackers. Instead, AI will act as a support tool streamlining workflows, surfacing insights, and allowing pentesters to focus on complex, logic-based exploitation and reporting.